Key words: Digital finance, financial inclusion, mobile money, digital banking, Zimbabwe
INTRODUCTION
Access to financial services is vital for poverty reduction and economic growth. Financial inclusion entails ensuring individuals and businesses have access to affordable financial products and services, including transactions, savings, credit, and insurance, delivered sustainably (Reserve Bank of Zimbabwe, 2022). It
"is defined as maximising access to and use of formal financial services while minimising barriers perceived by those outside the formal financial system (Cámara, Tuesta, & Vadillo, 2014, p. 1).
Financial inclusion fosters economic growth (Kim, Lee, & Newby, 2017) and resource mobilisation by promoting savings (Allen, Demirgüç-Kunt, Klapper, & Peria, 2012). However, globally, 65% of adults in developing nations lack access even to basic transaction accounts, let alone other services like savings, insurance, and credit (World Bank, 2020).
Digital financial services, driven by fintech, have the potential to lower costs, enhance transaction speed, security, and transparency, and offer tailored financial services, particularly benefiting the poor. The COVID-19 pandemic has underscored the importance of fintech in maintaining financial systems and ensuring safety during times of social distancing and economic uncertainty (World Bank, 2020). Despite the rapid growth in mobile phone and internet usage globally, over 2 billion people still lack internet access, and a third of the global population doesn't own a mobile phone (Hernandez, 2019).
However, there's a concern that a shift towards digital banking could exacerbate financial exclusion, particularly among low-income and technologically disadvantaged individuals (Ozili, 2017). The way digital finance is introduced in a country, voluntarily or forcibly, can also lead to voluntary financial exclusion if the population is not prepared for it. Hence, this study aims to explore the impact of digital finance on financial inclusion in Zimbabwe.
Financial inclusion has garnered significant attention since the early 2000s, with countries prioritising providing financial services to the previously unbanked population (Hanning & Jansen, 2008). The United Nations declared 2005 the International Year of Microcredit, highlighting the importance of financial inclusion (Siddik, Kabir, & Marathe, 2014). Over the past decade, there has been a global policy shift towards promoting greater access to finance, with key development institutions like the World Bank, International Monetary Fund (IMF), African Development Bank (AfDB), Asian Development Bank (ADB), and Organisation for Economic Co-operation and Development (OECD) dedicating substantial resources to understanding and promoting financial inclusion. Financial inclusion benefits include freedom from informal lenders, promoting economic growth and formalisation, poverty reduction, and financial stability (Reserve Bank of Zimbabwe [RBZ], 2022).
In 2017, nearly 1.7 billion adults worldwide (31% of the adult population) lacked access to financial institutions or mobile money providers. The majority of the unbanked were women and came from the poorest households (World Bank, 2022). In high-income OECD countries, only 6% of adults lack access to financial services, whereas in developing countries, about 80% of adults are financially excluded, with Sub-Saharan Africa having particularly high exclusion rates (Demirgüç-Kunt et al., 2015).
In Zimbabwe, 40% of adults do not have a bank account, and a significant portion relies on informal or non-formal financial services. Rural areas have the highest rates of financial exclusion, attributed to low income, cultural factors, and financial illiteracy (Mhlanha, 2021; Simbanegavi & Chivasa, 2016). Harare and Bulawayo urban areas exhibit lower rates of financial exclusion due to higher income levels, better financial literacy, and greater accessibility to financial institutions (Simbanegavi & Chivasa, 2016).
In Zimbabwe, the Reserve Bank promotes mobile money interoperability to drive financial inclusion, with digitisation playing a vital role during the COVID-19 pandemic (RBZ, 2022). The Reserve Bank of Zimbabwe (RBZ) has recognised the importance of financial inclusion and implemented the National Financial Strategy (NFIS) to drive inclusion. NFIS I focused on various themes, including improving access for women, youth, and people with disabilities, enhancing Micro, Small, and Medium Enterprises (MSMEs) access, promoting digital financial services, and improving microfinance (RBZ, 2020). NFIS II is currently being developed based on evidence from surveys conducted to assess the effectiveness of NFIS I strategies and to set targets for financial inclusion (RBZ, 2022). Figure 1 below shows financial exclusion in Zimbabwe.
Exclusion in Zimbabwe
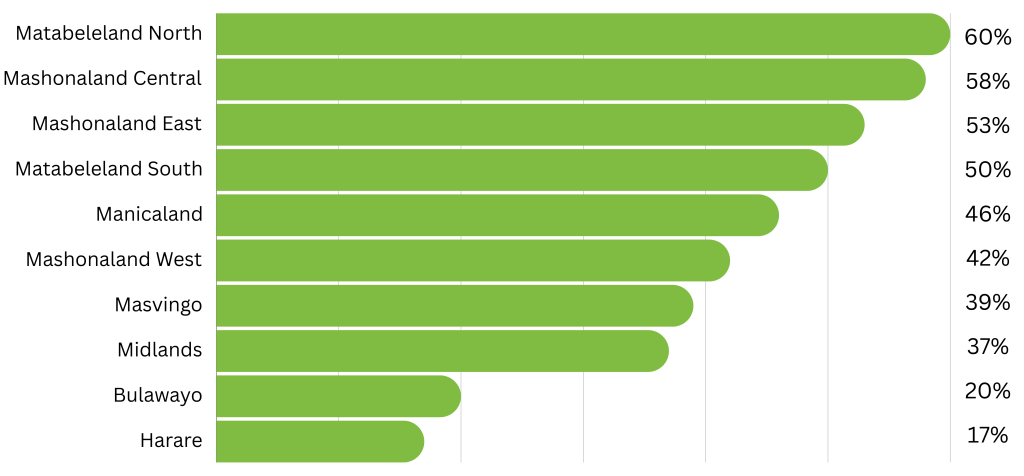
Figure 1: Financial exclusion by province in Zimbabwe
Source: Simbanegavi & Chivasa, (2016) Zimbabwe National Financial Inclusion Strategy (2016), Mhlanga, (2020a).
The Figure above shows that financial exclusion is largely concentrated in rural areas and the current status of Zimbabwe's financial sector does not seem to be promoting financial inclusion. Provinces such as Matabeleland North (60%), Mashonaland Central (58%), and Matabeleland South (53%) exhibit the highest rates of exclusion. These areas are predominantly rural, with limited access to essential services such as healthcare, education, and economic opportunities, which contributes to elevated exclusion levels.
Urban centres like Harare (17%) and Bulawayo (20%) report the lowest exclusion rates, likely due to improved access to resources and services commonly found in urban settings. This distribution underscores the contrast between rural and urban regions, with rural provinces experiencing greater challenges related to exclusion, influenced by factors such as geographical isolation and underdeveloped infrastructure.
Empirical evidence indicates that financially inclusive economies tend to record economic growth and a significant reduction in poverty (Beck, Demirgüç-Kunt, & Levine, 2007). However, the Sub-Saharan Africa (SSA) region has the greatest proportion of the population living in extreme poverty. It is the only region which has been recording an increase in poverty over the past two decades, with the poor getting worse off compared to other world regions (Statistical, Economic and Social Research and Training Centre for Islamic Countries, 2007; Simmons, 2015).
Access to financial services remains challenging for vulnerable groups worldwide, including low-income earners and the unemployed. These groups often face exclusion from the financial sector as institutions close branches in remote areas due to viability challenges (Mayo, Shaw, & Zhang, 1998). Kofi Annan emphasised the need to build inclusive financial sectors to uplift the poor (Agarwal, 2010, p. 2).
Despite efforts, poverty and inequality persist, prompting sustainable development initiatives prioritised by the United Nations (Arner, Barberis, & Buckley, 2020, p. 123). The 2030 Agenda for Sustainable Development outlines strategies toward peace, prosperity, and growth for all (United Nations, 2015, p. 15). Financial exclusion exacerbates deprivation, denying individuals appropriate access to financial services (Carbo, Gardener, & Molyneux, 2007, p. 98). In 2017, 31% of the global population lacked access to formal financial institutions, with 9% unbanked in the European Union (Demirgüç-Kunt, Klapper, Singer, Ansar, & Hess, 2018, p. 45).
Agufa (2016, p. 45) contends that digital finance in Kenya's banking sector lacks correlation with financial inclusion. Banks adopt digital services primarily to cut costs and enhance profitability, not to promote inclusion. The study reveals an insignificant negative relationship between agency, mobile, and internet banking with financial inclusion. Ozili (2018, p. 78) argues that digital finance by Fintech providers fosters financial inclusion in both emerging and advanced economies, especially for individuals with irregular incomes who find convenience more valuable than the higher costs associated with traditional banking. Kandpal and Mehrotra (2019, p. 92) suggest that customer confidence in traditional banking may hinder the adoption of new technologies, emphasising the importance of privacy and security in technological advancements. Durai (2019, p. 56) highlights the positive impacts of usability, convenience, and low service charges on mobile banking, mobile wallets, and credit cards, underscoring the significance of digital finance in enhancing financial inclusion. This study aims to evaluate the influence of digital financial innovation on financial inclusion in Zimbabwe, recognising the potential of these services to reach previously marginalised populations.
The rise of digital finance and FinTech offers hope for enhancing financial inclusion. These innovations transform the traditional financial sector into a more digital-driven one (Gomber, Koch, & Siering, 2017, p. 50). With 87% of the EU population already using digital payments and 47% accessing financial services via mobile or internet, digital finance and FinTech have the potential to broaden financial access and reduce barriers (Demirgüç-Kunt et al., 2018).
Digital innovations contribute to economic growth by bridging geographic distances and enhancing productivity through improved communication (Deloitte, 2007, p. 56; Chimhowu, Hulme, & Mkandawire, 2010, p. 224). Despite the potential benefits, some studies question the correlation between digital finance and financial inclusion (Agufa, 2016). However, others emphasise its positive impact, particularly in emerging economies (Ozili, 2018). Building customer confidence in traditional banking systems remains crucial for successful technology adoption (Kandpal & Mehrotra, 2019). Meanwhile, factors like usability and convenience drive the adoption of digital finance services (Durai, 2019). This study aims to explore the impact of digital financial innovation on financial inclusion, contributing to efforts to create more inclusive financial systems.
METHODOLOGY
The study employed a quantitative research design utilising econometric modelling. Quantitative methods were chosen for their ability to systematically collect, analyse, and interpret secondary time series data, which is essential for explanatory purposes in research (Creswell, 2003). The study focused on analysing time series data from 2000 to 2020, employing the fully modified ordinary least squares (FMOLS) method along with cointegration techniques to explore relationships over time.
The secondary data for the study was collected from established economic databases and statistical sources, ensuring reliability and validity in the analysis. Sampling methods were not applicable in the traditional sense since the study utilised existing datasets rather than collecting new data from a specific population or sample.
It's important to note that while the study had access to comprehensive secondary data sources, certain specific sources were unavailable, potentially impacting the comprehensiveness of the findings. This transparency is crucial for assessing the credibility and robustness of the study's conclusions.
Digital finance and financial inclusion model
The model assessed the impact of digital finance, specifically using automated teller machines (ATMs), as a proxy on financial inclusion. The selection of ATMs as a proxy for digital finance was based on their widespread adoption and influence in facilitating financial transactions globally. ATMs are a tangible indicator of digital financial infrastructure, enabling access to banking services in both urban and rural areas.
In addition to ATMs, the model included control variables such as gross domestic product (GDP), remittances, and broad money. These variables were chosen to capture broader economic and financial factors that could potentially influence the relationship between digital finance and financial inclusion. GDP reflects overall economic activity, remittances represent external financial flows, and broad money measures the total money supply within an economy.
While indicators like the number and value of mobile money transactions could also be valuable metrics for assessing digital finance's impact on financial inclusion, ATMs were specifically chosen due to their established role in traditional banking infrastructure and their accessibility across different regions. Future research could explore additional indicators to provide a more comprehensive analysis of digital finance's effects on financial inclusion, considering evolving technologies and consumer behaviours in financial services.
Descriptive statistics
Table 1 presents the descriptive statistics on financial inclusion, digital finance (ATM), GDP, remittance and broad money. Table 1 shows the mean, standard deviation, minimum and maximum values. From the descriptive statistics, it can be deduced that there is less variation between the mean and the median, standard deviation values are minimal and the Jarque- Bera statistics indicate that the data is normally distributed.
Table 1: Descriptive statistics
LFI | LBM | LATM | LGDP | RT | |
Mean | 1.364709 | 3.336929 | 1.805997 | 4.775232 | 5.533615 |
Median | 2.890429 | 3.166506 | 1.856298 | 4.540463 | 5.443556 |
Maximum | 3.291918 | 5.020909 | 2.071913 | 8.785335 | 13.61145 |
Minimum | -1.930708 | 2.658987 | 1.413423 | 4.043258 | 0.004048 |
Std. Dev | 2.115544 | 0.542536 | 0.202844 | 0.1101993 | 5.263840 |
Skewedness | -0.530329 | 1.628070 | -0.520035 | 2.759088 | 0.108320 |
Kurtosis | 1.460668 | 5.619260 | 1.908950 | 9.980748 | 1.349117 |
Jaque-Bera | 3.057719 | 15.28010 | 1.988117 | 69.28346 | 2.425804 |
Probability | 0.216783 | 0.000481 | 0.370072 | 0.000000 | 0.297333 |
Sum | 28.65888 | 70.07552 | 37.92594 | 100.2799 | 116.2059 |
Sum Sq. Dev | 89.51052 | 5.886910 | 0.822912 | 24.28775 | 554.1602 |
Observations | 21 | 21 | 21 | 21 | 21 |
Diagnostic test
Before conducting the main regression, the researcher did some diagnostic tests and these include: Unit Root Test (Augmented Dickey Fuller), Co-integration test, Autocorrelation Test and Multicollinearity test.
Normality test
Figure 2 below shows the normal distributions for the residuals. The Jarque- Bera probability value of 0.182910 is greater than 0.05, therefore we may fail to reject the null hypothesis that errors are normally distributed. This therefore means the errors are normally distributed with a mean zero and a constant variance.
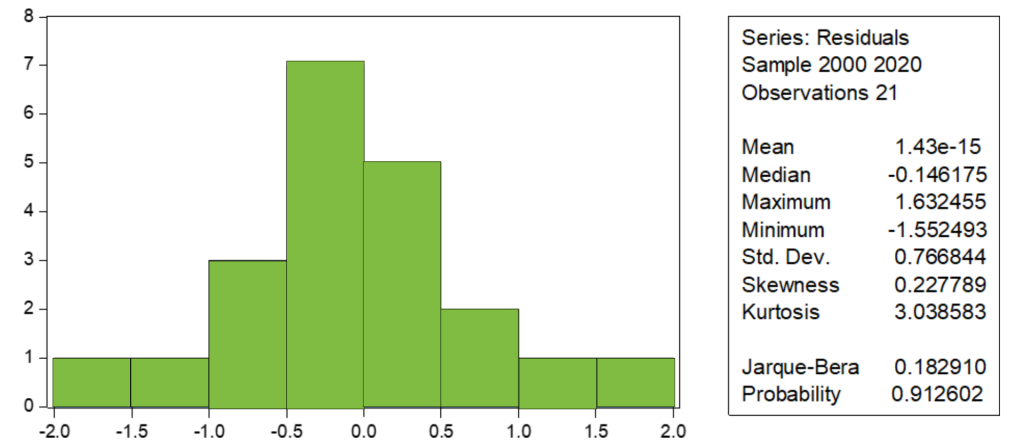
Figure 2: Normality graph
Source: Author's computations from Eviews version 12
Unit root test
This study utilised the Augmented Dickey Fuller test to assess variable stationarity. Gujarati (2004) indicates rejection of the unit root null hypothesis when the ADF statistic exceeds the critical value at different significance levels.
From Table 2 below, the results suggest that all the variables are not stationary at level. LBM, LATM and RT are stationary at first difference at intercept. However, all the variables are stationary at the second difference at both intercept and at intercept and trend.
Table 2: Unit root test
Variable | Level Intercept Intercept and Trend | First Difference Intercept Intercept and Trend | Second Difference Intercept Intercept and Trend | |||
LFI | 0.3851 | 0.6684 | 0.4480 | 0.5965 | 0.0081 | 0.00334 |
LATM | 0.0800 | 0.2823 | 0.0446 | 0.1364 | 0.0004 | 0.0025 |
LBM | 0.2300 | 0.4056 | 0.0032 | 0.0154 | 0.0015 | 0.0096 |
LGDP | 1.0 | 0.3706 | 0.4761 | 0.9972 | 0.0832 | 0.0985 |
RT | 0.5242 | 0.8032 | 0.0040 | 0.0177 | 0.0000 | 0.0001 |
Table 3: Unit root test
Variable | ADF Statistics | Test Critical Value | P-Value | Order of Integration |
LFI | -3.963301*** | 1% -3.857386 | 0.0081 | I(2) |
5% -3.040391 | ||||
10% -2.660551 | ||||
LATM | -5.505888*** | 1% -3.857386 | 0.0004 | I(2) |
5% -3.040391 | ||||
10% -2.660551 | ||||
LBM | -4.841801*** | 1% -3.886751 | 0.0015 | I(2) |
5% -3.052169 | ||||
10% -2.666593 | ||||
LGDP | -2.764356* | 1% -3.857386 | 0.0832 | I(1) |
5% -3.040391 | ||||
10% -2.660551 | ||||
RT | -7.483253*** | 1% -3.857386 | 0.0000 | I(1) |
5% -3.040391 | ||||
10% -2.660551 | ||||
RESID | -5.5666101*** | 1% -4.004425 | 0.0007 | 1(1) |
5% -3.098896 | ||||
10% -2.690439 |
The results from the Table 3 above show that all the variables are stationary at second difference with the order of integration I(2) at all significant levels that is 1%, 5% and 10% respectively as indicated by the three asterisks except LGDP which is stationary at first difference at 10% significance level.
Co-integration results
The co-integration test was done in order to circumvent the problem of spurious results. This was done by conducting a unit root test for residuals generated. The results for model one revealed that residuals are stationary after the second differencing which signifies that there is a long-run relationship between digital finance and financial inclusion since the ADF statistic 5.5666101 is greater than the critical value at all levels of significance: 1%, 5% and 10% (see table 3).
Autocorrelation
The Durbin-Watson (DW) statistic was utilised by this study to determine whether the model is safe from a first order serial correlation. In OLS if it is present this will overestimate R squared and simultaneously makes t and F tests invalid hence likely to lead to spurious results. This is the case when R squared is significantly greater than DW. The null hypothesis is stated as: there is no autocorrelation against the alternative of autocorrelation among residuals. The LM test results show a DW value of 1.914167 which falls within the rejection region of the null hypothesis.
The above mentioned DW statistics results is greater than 1.5 as a rule of thumb, and also the p- values are significantly large and greater than 0.05 showing the evidence of no serial autocorrelation among the residuals and hence we fail to reject the null hypothesis.
Multi-collinearity
This is the presence of a linear relationship among the explanatory variables. As a result of the stochastic nature of most explanatory variables correlation and interrelationships might exist. It has the effect of making the equation indeterminate. When multi-collinearity is there the remedy is to drop the variable. Table 4 below shows the zero-order correlation co-efficient of the explanatory variable.
Table 4 shows that the pair-wise correlation co-efficient for all the independent variables is less than 0.8, as a rule of thumb, with the exception of RT and LFI. It can therefore be concluded that multi-collinearity is not severe since it is less than 0.8. Therefore, the explanatory variables do not exhibit serious correlations or relationships amongst them. This means that there is no linear relationship among the explanatory variables and it is easy to establish the influence of each one variable on the dependent variable.
Table 4: Correlation matrix (explanatory variables)
LFI | LBM | LATM | LGDP | RT | |
LFI | 1.000000 | -0.448822 | 0.161322 | 0.465551 | 0.863241 |
LBM | -0.448822 | 1.000000 | 0.380701 | -0.312840 | -0.550762 |
LATM | 0.161322 | 0.380701 | 1.000000 | 0.092864 | -0.112803 |
LGDP | 0.465551 | -0.312840 | 0.092864 | 1.000000 | 0.239739 |
RT | 0.863241 | -0.550762 | -0.112803 | 0.239739 | 1.0000000 |
The strength and direction of the linear relationship between two variables was measured by performing a correlation matrix. The correlation test shows a positive relationship of 0.161322 between financial inclusion (LFI) and digital finance (LATM). GDP positively correlated to financial inclusion with 0.465551. Broad money (LBM) is negatively correlated with financial inclusion with a coefficient of 0.448822 and finally remittances (RT) is positively correlated with financial inclusion with a coefficient if 0.863241.
To this end, the researcher adopted the do-nothing school of thought as expressed by Blanchard (1967) in Gujarati (2004). This means that there is no linear relationship among the explanatory variables and it is easy to establish the influence of each one variable on the dependent variable, financial inclusion separately. The strength and direction of the linear relationship between two variables was measured by performing a correlation matrix.
Heteroskedasticity
The Bruesch-Pagan Godfrey Test probability value of 0.2993 is greater than the significance level of 0.05. This therefore implies that the errors are homoscedastic and that we may fail to reject the null hypothesis that the errors are homoscedastic.
REGRESSION RESULTS
The researcher regressed time series data as per the model specified. The regression results are presented in Table 5.
Significance and fitness of the whole model is revealed by the F-statistic and the explanatory power predictability capacity of the independent variables shown by R squared (the coefficient of determination). The Table 5 above shows model one regression results, the R-squared is 0.838641 thereby showing that about 84% of variations in financial inclusion are explained by the combined variations in the explanatory variables. However, the R-squared statistic cannot really exhibit a true explanation that variations in financial inclusion are explained by combined variations in explanatory variables mainly because when we increase the number of explanatory variables R-squared value will continue to go up even if we add unrelated explanatory variables hence there is need to consider the adjusted R-squared. The adjusted R-squared is at 0.795613 thereby showing that indeed about 80% of variations in financial inclusion are explained by combined variations in the explanatory variables used in this study and the remaining 20% can be credited to other stochastic factors. Since the adjusted R-squared is close to R-squared, we may conclude that the model is a good fit since more than half of the variations in the dependent variable are explained within the model.
It can be deduced from the regression results that LATM, LGDP and RT are significant. LATM a proxy for digital finance exhibits (with a coefficient of 3.116906) a positive strong significant relationship with financial inclusion. This means that a unit increase in digital finance will result to 3.116906 increase in financial inclusion. Meanwhile, a unit increase in gross domestic product proxied by LGDP will result in 0.464005 increase in financial inclusion (LFI). Furthermore, remittances (RT) show a positive relationship with financial inclusion (LFI) and thus a unit increase in remittances will results in 0.369439 increase in financial inclusion.
On the other hand, broad money (LBM) a proxy for financial development depicted a positive relationship with financial inclusion and it’s highly insignificant and thus a unit increase in financial development (broad money) will result in 0.039869 insignificant increase in financial inclusion. The significance of variables is measured by the t- statistic values and the P values. A variable is said to be significant if its absolute t-statistic is greater than two or a neighbour of 2 and/or if its p-value is less than 0.05. From the results three variables are significant in explaining variation in financial inclusion in Zimbabwe for the period 2000 to 2020, that is, digital finance (LATM), GDP and remittances. Digital finance (LATM), gross domestic product (LGDP) and remittance (RT) have t-statistics greater than 2 and p-values less than 0.05 respectively. The focus of the study is on the coefficient of the proxy of digital finance (LATM) which reflects a positive and significant relationship with financial inclusion.
Table 5: Regression results - fully modified least squares
Variable | Coefficient | Std. Error | T-Statistic | Probability | |
LBM | 0.039869 | 0.319012 | 0.124978 | 0.9022 | |
LATM | 3.116906 | 0.793673 | 3.927193 | 0.0013 | * |
LGDP | 0.464005 | 0.125435 | 3.699161 | 0.0021 | * |
RT | 0.369439 | 0.030092 | 12.27715 | 0.0000 | * |
C | -8.672733 | 1.613921 | -5.373704 | 0.0001 | |
Significant | 1%* | 5% ** | 10%*** |
R-Squared | 0.838641 | Mean Dependent Var | 1.529479 |
Adjusted R-Sqaured | 0.795613 | S.D. Dependent Var | 2.027544 |
S.E of Regression | 0.916637 | Sum Squared Resid | 12.60335 |
Long Run Variance | -0.323410 |
DISCUSSION
The study utilised the Cointegration technique to analyse the relationship between digital finance and financial inclusion in Zimbabwe from 2000 to 2020. The findings indicate a positive association between digital financial innovation and financial inclusion. Specifically, a unit increase in digital financial innovation corresponds to approximately a 3.116906 unit increase in financial inclusion. This aligns with expectations and is supported by similar research. Borges (2020) examined the impact of digital financial services on financial inclusion in Mozambique, finding a positive relationship using the Autoregressive Distributed Lag (ARDL) model. The study differentiated between traditional and innovative digital payment methods, concluding that innovative methods contribute significantly to financial inclusion.
Ozili (2018) corroborates these findings, suggesting that digital finance, particularly through fintech providers, enhances financial inclusion and stability in both emerging and advanced economies. This is attributed to the convenience digital finance offers, especially to individuals with low and variable incomes. Similarly, Widarwati, Solihin, and Nurmalasari (2022) found a positive impact of digital finance, measured by Average Digital Finance (ADF), on financial inclusion in Indonesia. They emphasise the necessity of digital finance breakthroughs to optimise financial assistance and improve inclusion, highlighting the role of technological support.
Andrianaivo and Kpodar (2012) established a significant positive relationship between mobile phone penetration and financial inclusion, emphasising the role of mobile technology in providing cost-effective financial services to the underserved.
Domeher et al. (2022) further support this, identifying a positive nexus between financial innovation and inclusion. Additional studies by Durai and Stella (2019), Han and Du (2021), and Mutua (2018) also confirm the positive relationship between digital finance and financial inclusion. However, some dissenting voices, like Agufa (2016), argue that digital financial innovations primarily aim to reduce operational costs and improve profitability, rather than foster inclusion.
Generally, the collective body of research suggests a strong positive correlation between digital finance and financial inclusion. While dissenting opinions exist, the majority of studies support the notion that digital financial innovation plays a pivotal role in enhancing access to and usage of financial services, ultimately leading to greater financial inclusion.
CONCLUSION
Given the findings of this research, the following conclusions can be drawn:
The relationship between digital financial innovation and financial inclusion
The study reveals a strong positive correlation between digital financial innovation and financial inclusion in Zimbabwe. Digital finance adoption has risen due to its perceived usefulness and ease of use. Stakeholders, especially banks and the Reserve Bank of Zimbabwe (RBZ), should invest in robust digital finance infrastructure. Supporting digital financial innovation is crucial as it drives financial inclusion. Access to digital finance products like mobile banking, internet banking, agency banking, mobile money, and ATMs has enhanced financial inclusion. Thus, fostering the growth and accessibility of these services is key to promoting greater financial inclusion in Zimbabwe.
Usage of digital finance and financial inclusion
The study shows a significant positive correlation between digital finance and financial inclusion in Zimbabwe. Increased use of mobile banking, internet banking, and agency banking enhances financial inclusion. These digital products are cheaper, reliable, and convenient, supporting financial intermediation theory. Stakeholders should invest in digital financial literacy to promote inclusion.
RECOMMENDATIONS
The research proposes several recommendations to enhance financial inclusion in Zimbabwe:
Increase Security Measures: Establish robust cybersecurity mechanisms to safeguard digital financial services against fraud. Government intervention, through the Reserve Bank of Zimbabwe or related ministries, should ensure investment in cybersecurity infrastructure to protect digital innovations.
Introduce Access to Loans via Digital Platforms: Enable loan access through digital platforms like mobile banking for financially excluded individuals. Collaborations between mobile network operators and financial institutions can facilitate soft loans via mobile banking.
Group Schemes Integration: Design digital platforms to accommodate group schemes, encouraging savings and digital banking usage among communities, particularly low-income earners, rural populations, and marginalised groups.
Address Barriers to Financial Inclusion: Banks and regulators should tackle barriers such as financial illiteracy. Empowering individuals with financial knowledge and skills can significantly improve financial inclusion.
Promote Technological Innovation: Embrace technological innovation, such as branchless banking and mobile banking, to reach the unbanked population. The government should support this with a conducive legal framework.
Promote Infrastructure Development: Invest in infrastructure development in rural and marginalised areas to improve accessibility for financial services. Government support and incentives for private sector investment in ICT infrastructure are crucial.
Promote Increased Cooperation among Banks: Encourage collaboration and infrastructure sharing among banks to spread the cost of technology for financial inclusion initiatives. Establishing entities for pooled resources, such as Special Purpose Vehicles, can facilitate shared infrastructure usage.
REFERENCES
Agarwal, A. (2010). Kofi Annan and the need for inclusive financial sectors. Global Development Press.
Agufa, M. (2016). The impact of digital finance on financial inclusion: A case of Kenya’s banking sector. Journal of Economic and Financial Studies, 3(2), 40-60.
Allen, F., Demirgüç-Kunt, A., Klapper, L., & Peria, M. S. M. (2012). The foundations of financial inclusion: Understanding ownership and use of formal accounts. World Bank Policy Research Working Paper, 6290, 7-39.
Beck, T., Demirgüç-Kunt, A., & Levine,
R. (2007). Finance, inequality, and the poor.
Journal of Economic Growth, 27-49.
Borges, T. (2020). Digital financial services and financial inclusion: Evidence from Mozambique using the ARDL model. Journal of Financial Inclusion Studies, 15(2), 45-60.
Cámara, N., Tuesta, D., & Vadillo, S. (2014). Financial inclusion in Latin America and the Caribbean: Review and new agenda. Inter- American Development Bank.
Chimhowu, A., Hulme, D., & Mkandawire, T. (2010). The role of innovation in Africa's development: Financial inclusion through digital technology. African Development Review, 22(2), 220-235.
Creswell, J. W. (2003). Research design: Qualitative, quantitative, and mixed methods approaches. SAGE Publications.
Deloitte. (2007). Economic impacts of digital finance: Bridging the divide. Deloitte Insights.
Demirgüç-Kunt, A., Klapper, L., Singer, D., Ansar, S., & Hess, J. (2018). The Global Findex database 2017: Measuring financial inclusion and the fintech revolution. World Bank.
Domeher, D., Saka, K., & Frimpong, J.
M. (2022). (2022). Financial innovation and inclusion in emerging markets. Journal of Banking and Finance, 56, 1-20.
Durai, T. (2019). The impact of mobile banking on financial inclusion: Evidence from developing economies. International Journal of Business Management and Economic Research, 10(1), 50-65.
Durai, T., & Stella, R. (2019). Digital finance: Enhancing financial inclusion in developing countries. Finance and Development, 10(1), 45-65.
Gomber, P., Koch, J.-A., & Siering, M. (2017). Digital finance and fintech: Current research and future research directions. Journal of Business Economics, 87(5), 537-580.
Gujarati, D. N. (2004). Basic econometrics (4 ed.). McGraw-Hill.
Hernandez, K. (2019). Global perspectives on internet access and mobile phone ownership: The digital divide persists. Digital Development Research, 7(3), 45-58.
Kandpal, V., & Mehrotra, R. (2019). Financial inclusion: The role of fintech and digital financial services in India. Indian Journal of Economics & Business, 19(1), 85-93. https://ssrn.com/abstract=3485038
Kim, D. W., Lee, S. C., & Newby, T. (2017). The impact of financial inclusion on economic growth: A global perspective.t,. Journal of Business Economics and Management, 18(1), 61-76.
Mayo, E. S. (1998). The viability of rural financial institutions in a digital age. Rural Finance Research Serie, 5(3), 45-78.
Mhlanga, D. (2021). Factors that matter for financial inclusion: Evidence from Sub- Saharan Africa.The Zimbabwe case. Journal of African Development, 10(6), 112-129.
Mhlanha, L. (2021). Financial inclusion and the informal sector in Zimbabwe. Journal of Economic Policy Research, 9(2), 23-37.
Ozili, P. K. (2018). Impact of digital finance on financial inclusion in developing countries. Journal of Financial Regulation and Compliance, 26(2), 76-84.
Ozili, P. K. (2017). Financial technology and financial inclusion: A systematic review. 55(3), 311-342.
Reserve Bank of Zimbabwe. (2020). National Financial Inclusion Strategy 1. Reserve Bank of Zimbabwe, 2016-2020.
Reserve Bank of Zimbabwe. (2022). Annual report on financial inclusion in Zimbabwe. Harare: Reserve Bank of Zimbabwe.
Reserve Bank of Zimbabwe. (2022). Monetary policy. Harare: Reserve Bank of Zimbabwe.
Siddik, M. N. A., Kabir, M. A., & Marathe, S. K. (2014). Financial inclusion and microcredit: The role of financial institutions in promoting access. Journal of Economic Development, 39(3), 1-22.
Simbanegavi, S., & Chivasa, P. (2016). Financial inclusion in Zimbabwe post- hyperinflationary period: Barriers and effects on societal livelihoods, a qualitative approach. Case study of Matebeleland North. Journal of Financial Stability, 5(4), 333-350.
Simmons, R. (2015). Poverty and inequality in Sub-Saharan Africa. International Journal of Development Issue, 14(1), 3-21.
Statistical, Economic and Social Research and Training Centre for Islamic Countries. (2007). The poverty and inequality challenge in Sub-Saharan Africa. SESRIC.
United Nations. (2015). The 2030 Agenda for Sustainable Development. United Nations.
Widarwati, A. A., Solihin, R., & Nurmalasari, I. (2022). The impact of digital finance on financial inclusion: A study of Indonesia. Asian Journal of Economic and Financial Studies, 10(2), 33-49.
World Bank. (2020). Digital financial services. Global Financial Development Report.
World Bank. (2020). The Global Findex database 2020: Measuring financial inclusion and the fintech revolution. World Bank.
World Bank. (2022). The Global Findex database 2021: Financial inclusion, digital payments. World Bank.